Listen to this article:
You know what they say in Silicon Valley: GenAI or die.
That’s a bit of an oversimplification, but the sentiment is real. These days a tidal wave of VC money is going to startups that incorporate generative artificial intelligence, or GenAI. Series A companies are also feeling the pressure: Those that don’t lean into GenAI are having a harder time securing second- or third-round funding. Whatever sector you’re in, and whatever stage of your business, if you’re a founder or CEO seeking VC backing at this moment, you’re expected to harness this emerging technology.
OpenAI made ChatGPT-3 available to the general public in November of 2022, and since then the new generation Large Language Model (LLM) has dominated the discourse. Further complicating the issue is the fact that it’s not just trendy. It’s also truly revolutionary. But for founders and funders alike, cutting through the fanfare to glean a clear view has proven exceedingly difficult. For evidence of the confusing state of the market, look no further than Databricks, which spent $1.3B to buy LLM-software company MosaicML in June, then announced a $380M loss in August in a call for more funding.
Then there’s the recent valuation of Hugging Face, a public AI-model repository, at a mind-bending $4.5B after it received $235M in fourth-round funding from the likes of Google, Amazon, Salesforce and Nvidia. That number is huge—and in the GenAI sector, they’re only getting huger.
The AI-money landscape is surreal enough that some VCs are resigned to humor as the only way to explain it. Founders, CEOs and entrepreneurs who don’t have a spare mil lying around are rightfully fascinated by the field and/or compelled to join it for the sake of survival. All of us could benefit from a sober assessment of where things stand. What follows is mine. And though I’m backed by two decades of launching in Silicon Valley and a decade of hands-on building with AI and ML, I’m always up for healthy debate.
Plot the curve
Let’s start by plotting GenAI’s position in the hype cycle vis-a-vis its long-term market potential. Personally, I try to temper my inherent techno-optimism with a healthy dose of techno-pragmatism.
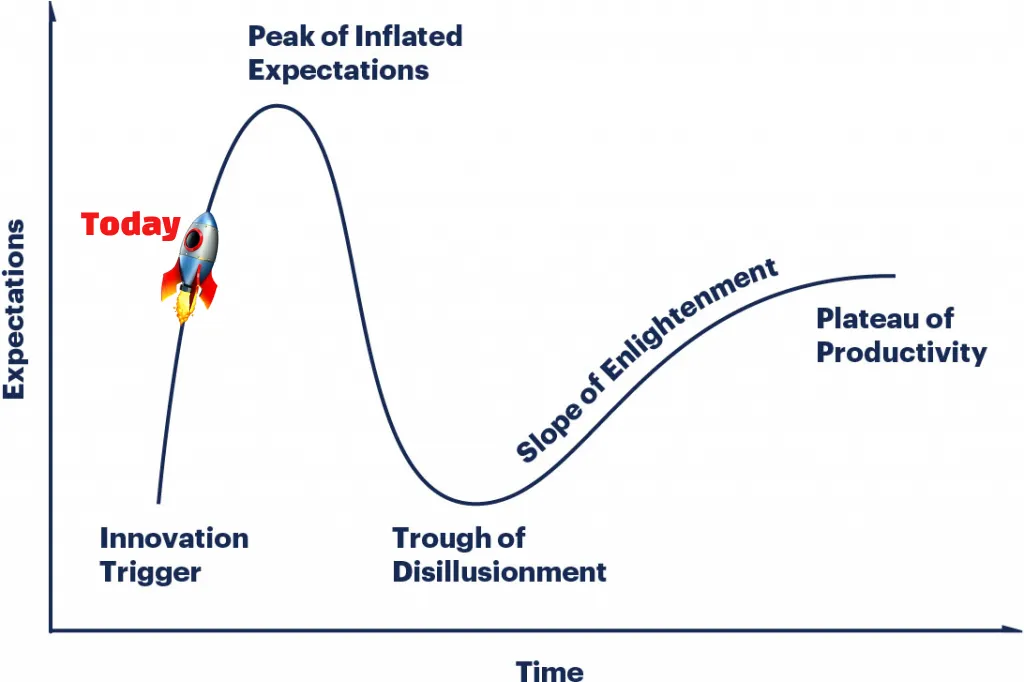
In my estimation, GenAI hasn’t even hit the Peak of Inflated Expectations yet. But ultimately it’s still too new to say. I think most people agree. At this point, comparison is more useful than speculation.
Suppose GenAI is as much a once-in-a-generation crux as the advent of the Internet. Many observers have suggested as much, including no less an expert than Bill Gates.
Let’s recall that similarly lofty claims have been made about blockchain.
Despite the resources poured into advancing blockchain and crypto, they remain mired in the Trough of Disillusionment. Credit card transactions are faster than crypto, Venmo is faster than crypto, and crypto’s privacy is not as impenetrable as was once believed. It will likely never replace money. It isn’t a failure, but it hasn’t lived up to the hype that surrounded it.
The Internet, however, is doing fine. After the dotcom crash of the early 2000s—its nadir in the Trough—it climbed to the Plateau of Productivity and has been cruising there for a while now. Once the world was enabled to buy shoes and read news online, it wasn’t going back to print catalogs or newspapers.
So GenAI: Is it the Internet? Or is it blockchain?
Just about every possible use case for GenAI that I’ve run through, even casually, is a potential game-changer. It’s capable of exponentially improving processes across medicine, engineering, logistics and countless other sectors, and many others that we haven’t yet imagined.
Jeff Bezos was once asked for his predictions of what will happen in the next 10 years. He responded instead with what he knows won’t happen in the next ten years: Amazon customers will not want slower shipping, and they will not want more expensive shipping. Now that it’s cheap and quick there’s no going back. “When you have something that you know is true, even over the long term,” he said, “you can afford to put a lot of energy into it.”
Once we have faster, more accurate MRI analyses, cancer diagnoses and gene therapies, we will not want slower ones. We’re not going back. We’re seeing a lot of energy being put into GenAI, and that’s because we know its improvements to be true. And they’re only expanding over the long term.
Take a look at your own business model. What is the long-term truth at its core? What is your forever thing? That is your anchor amid the waves of hype and whirlwinds of cash.
Audit your resources
Founders, you should indeed be nurturing a relationship with GenAI in some shape or form at this very moment. But when it comes to expectations, be pragmatic. The landscape is still foggy. Cost to adopt is high (more on that in a minute), ROI is as tricky as ever and, in the end, your current business structure may not be ready for it.
The good news is most businesses have enough proprietary data to justify embracing GenAI. The key is assessing your resources with an open mind.
Loka is currently working with a company that operates in an industry well outside what the perceived standards for GenAI adoption. But through a very creative understanding of their users and their needs, they’re engaging in a few rather novel applications for the software. They don’t need GenAI per se, but GenAI is moving them forward dramatically.
Indeed, you might surprise yourself. If not with your own proprietary data then with the opportunities afforded by combining open-source data repositories and machine learning tools. Start by abandoning old formulas and applying left-field thinking.
Sticker shock?
ChatGPT and LLM tools that are mesmerizing the tech world don’t come cheap. But their benefits can be invaluable. Loka has been deploying AI and ML for our clients for a long time, so we have a clear idea of the practical considerations at stake. What are the true costs of switching on GenAI and maintaining an LLM? My goal in demystifying this process is to shed light and set expectations, not to scare anyone away.
Computing Cost
The engine of the computer or phone you’re reading this article on is a central processing unit. The CPU is an all-purpose processor that’s powerful and efficient. Generative AI, including LLMs and natural-language image generators, run best on graphics processing units. GPUs are more specialized than CPUs, capable of performing certain operations much more efficiently. They’re also much more power-hungry.
GPUs are in incredibly high demand right now, as you can probably imagine. Which helps explain why Nvidia, the company responsible for building 95% of GPU chips on the market, recently reported $6 billion in pure profit on a staggering $13.5 billion in revenue. That’s $6 billion quarter over quarter, not year over year. This is Apple-level profitability, as rare as it gets.
For founders, the important thing to think about is that higher compute cost translates into a higher financial cost, as we’ll see below.

Server Cost
ChatGPT posts its pricing plans on its website. This information is so granular that it’s hard to extrapolate into use-case-budgeting terms. When it comes to the big picture, Google and OpenAI are opaque with financial information. How much does it cost to train an LLM? When asked if it cost $100 million to train ChatGPT, founder Sam Altman answered with a vague, “It’s more than that.” In other words, it’s very expensive. Probably very, very expensive. But with valuations and profits already so insanely high, even “very, very expensive” is relative.

What we do know is that GenAI costs are affected by many variables and fluctuate wildly. They follow not only macrotrends like compute costs and chip costs, but microtrends that occur on a minute-to-minute basis. The more people using ChatGPT or LLAMA-2 at any particular moment, the cheaper its operating costs.
Another example I can share is the cost of Loka’s real-time endpoint on AWS’s SageMaker platform. We pay $12,000 a month for self-hosting, meaning we pay for the server space, not what's running on it. Costs may come down if you opt to share a server with multiple customers, ie. cost is divided by the number of users. We pay for the best LLM available from Meta, called LLAMA-2, which accommodates 70 billion parameters, and run it on a single machine. It’s heavy stuff. Other applications don’t require that kind of compute.
Keep an eye out for AWS’s new serverless model called Bedrock, which is rolling out as we speak. We have early access to the product, and from what we’ve seen, it’s less expensive, and yet another game-changer.
One thing to keep in mind is that this is our monthly cost–not our total maintenance. We have our own internal team that optimizes spend. Do you have the team to maintain an LLM? How will your usage fluctuate? Once it’s on, does the ROI pencil?
The big picture
Most VCs I know investing today remind me that they expect liquidity in 7-10 years. If that seems like a long time, consider that billion-dollar IPOs Airbnb, Doordash and Snowflake—let’s call them the Class of 2020—were all launched at least 10 years earlier. Building a successful tech company remains a marathon, not a sprint. But to overextend the metaphor, you can expect water stations along the route where you can get a boost. And the goal isn’t to win the next leg, but the entire race.
Right now the smart bet is on balance, not blockbuster. Slow and steady is not the typical startup pace, but it’s important to be patient in the midst of a hype storm. We build out of this moment not with all-in bets but with well-paced, incremental steps. We take them not only with courage and excitement but clear eyes about where we’re heading.
Three takeaways
1. Data moats are the news moats. In a business context, a moat is a company’s defense against competition, the thing that differentiates you in the market. (Thank Warren Buffett for the analogy.) When you’re considering GenAI, your data is your moat. What data are you collecting—or could you collect—that's yours alone, hard for others to mimic? Any LLM is only as powerful—and as unique—as the data it’s trained on.
2. Be creative. Assuming you’re not Google or Netflix or some other company collecting a treasure trove of proprietary data every second, you still have thousands of publicly available data sets that you can add to your paint set. You aren’t collecting data to use or sell externally, you’re collecting it for your own internal use.
3. Find your focus. Running LLMs is expensive. Make sure your upside is working toward your equivalent of Bezos’ vision of two-day shipping today, one-hour shipping tomorrow. As a founder or CEO, at the end of the day, only you know where you are with your business. Armed with that knowledge, your experience and the advice above, you can build a roadmap that determines when and where you connect with GenAI and how deep you dive in.