Listen to this article:
Artificial intelligence is leading a new era of innovation, making drug discovery faster, more efficient and more accessible. Conversational AI tools like ChatGPT and Claude dominate the popular consciousness, but specialized applications of generative AI such as biological large language models (BioLLMs) are driving true breakthroughs, particularly in healthcare and life sciences. Once expensive, time consuming and complex to use, these tools are now more widespread than ever.
Loka is at the forefront of this revolution, positioned at the intersection of AI, healthcare and life sciences. In the last two years, we’ve worked with a dozen clients to harness the power of BioLLMs to help tackle cancer, liver disease and other widespread afflictions. The results are already transforming medicine on the way to saving lives.
Seeing the Future in HCLS
We’re not the only ones who see tremendous real-world impact on the near horizon. With a similar appreciation for current and potential results, the 2024 Nobel Prize in Chemistry went to groundbreaking AI researchers who are transforming drug discovery.
The Nobel academy doesn’t recognize a computer science category, but that didn’t stop them from honoring a group of computer scientists who work in biochemistry: Demis Hassabis and John Jumper from Google DeepMind and David Baker from the University of Washington. Hassabis and Jumper were recognized for developing AlphaFold, an AI model that can predict the 3D structures of any protein sequence with high accuracy, solving a decades-old problem. Baker was honored for his work in designing novel proteins from scratch using computational methods, including the development of new protein structures with tailored functions. His research opens up new avenues for therapeutic development, including the creation of custom enzymes and disease-targeting proteins. These two achievements have profoundly impacted fundamental research and opened new frontiers in drug discovery.
BioLLMs have led to major recent breakthroughs in HCLS. These LLMs are specifically designed to understand biological sequences and data in a way similar to the way they understand natural language. Models such as ESM [1], MegaMolBART [2], and DNABERT [3] learn representations for proteins, small molecules and DNA, enabling a wide range of prediction and generation tasks. By harnessing the power of BioLLMs and integrating them into the drug development process, companies and labs can accelerate the pace of drug discovery.
Developing a new drug is a rigorous process that historically takes 10-15 years and costs around $2 billion per successful drug brought to market (Figure 1). More than 95% of drug development programs fail due to factors like toxic side effects or lack of efficacy in the clinical stages. The early stages—target identification, lead identification/screening and lead optimization—are especially costly and time-consuming due to the sheer number of biomolecules that need to be tested. In fact, for every 10,000 compounds screened, only 1-2 may eventually make it to clinical trials.

AI, and BioLLMs in particular, are revolutionizing early drug discovery by both expanding and refining the pool of potential drug candidates and therapeutic targets. GenAI models such as MegamolBART for small molecules enable de novo generation of compounds and exploration of new molecular structures, thus increasing candidate diversity. For proteins, models like ProtGPT2 [4] and ProGEN [5] enable the de novo generation of new proteins with tailored characteristics and the design of proteins with novel properties. Simultaneously, predictive AI models, including ProtT5 [6] and ESM for proteins, Molformer [7] for small molecules and DNABERT and HyenaDNA [8] for nucleic acids, can predict structural and functional traits as well as characteristics like solubility and affinity. However, the true strength of these models lies in their ability to be fine-tuned to specific tasks, allowing experts to tailor them to address their needs.
These models filter out low-potential leads so researchers can focus on the most promising candidates, reducing the need for extensive wet-lab testing. Integrating these models not only accelerates development and cuts costs but also overcomes laboratory constraints by shifting limitations to budget and computational capacity. This approach significantly increases the number of molecules that can be screened and tested while improving the evaluation of their therapeutic potential (Figure 2).
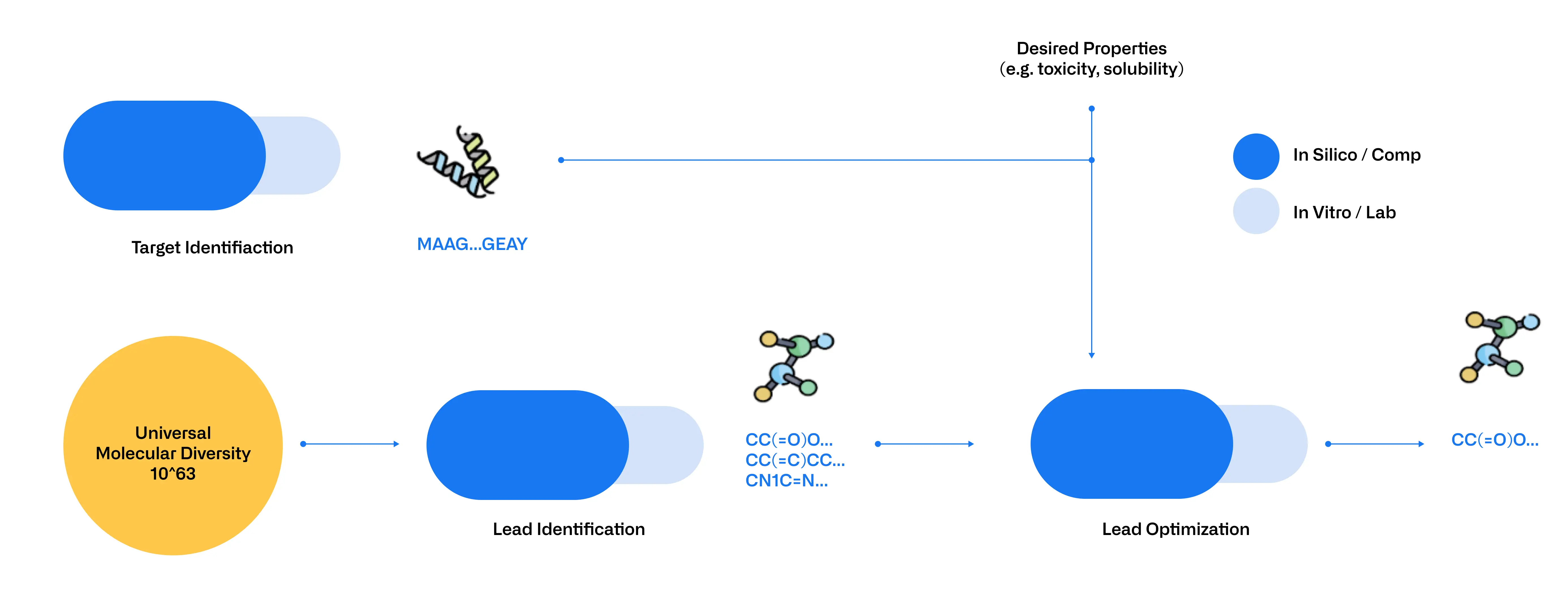
The Inner Workings of Bio LLMs
Proteins, DNA and small molecules can be viewed as “languages” because they’re composed of sequences of fundamental building blocks. For example, the “language” of proteins consists of 20 amino acids. Each protein is formed by arranging these amino acids in a unique sequence, and this specific combination defines the protein’s structure and, consequently, its function. Thus, different amino acid sequences create different proteins, each with a distinct role in biological processes.
Just as general LLMs such as ChatGPT have transformed the way machine learning is applied to natural language, BioLLMs are transforming the way machine learning is applied to biological data by learning to understand and “speak” in biological languages.
There are three important ingredients that are crucial for the success of LLMs in general and BioLLMs in particular:
- Abundant Data. New bioinformatics and experimental methods such as high-throughput sequencing are generating more data than ever, which typically is not annotated.
- State-of-the-art Deep Learning.Neural network architectures such as transformers, the model behind large language models (LLMs), are designed to focus on different parts of a sequence using attention mechanisms. They scale effectively, enabling the training of large models on massive datasets, and excel at capturing long-range relationships, such as those found in biological sequences.
- Self-Supervised Learning. This modeling paradigm allows learning from unlabeled data by asking the model to predict masked parts of the sequence.
By accelerating the identification and optimization of drug candidates, BioLLMs demonstrate how much of the research process can now be performed in silico, with wet-lab experiments serving primarily for validation. This shift supports a bold vision for the future—one where AI is not just a tool for drug discovery but becomes its central architect. Nobel winner Demis Hassabis suggested a reorganizing of this old, expensive process by asking, “What would drug discovery look like if you approached it from an AI-first perspective, rather than as an add-on?”[9]
Bioengineers at Loka are committed to making this vision a reality. By helping companies reengineer their drug discovery pipelines, we integrate GenAI to dramatically accelerate development of new therapies, enabling faster delivery of life-saving treatments to patients. Keep an eye out for Part 2 on this topic, where we’ll get more technical and share practical examples of how we are working with clients to achieve these goals and deliver tangible results.
To learn more about Loka’s BioLLM services, visit Loka's BioLLMs page or contact Jorge Sampaio at jorge.sampaio@loka.com or Telmo at telmo@loka.com.
References
- [ESM] https://github.com/facebookresearch/esm
- [MegaMolBART] https://github.com/NVIDIA/MegaMolBART
- [DNABERT] https://github.com/jerryji1993/DNABERT
- [ProtGPT2] https://huggingface.co/nferruz/ProtGPT2
- [ProGen] https://github.com/salesforce/progen
- [ProtT5] https://huggingface.co/Rostlab/prot_t5_xl_bfd
- [MolFormer] https://github.com/IBM/molformer
- [HyenaDNA] https://github.com/HazyResearch/hyena-dna
- https://endpts.com/exclusive-deepmind-ceo-demis-hassabis-talks-secretive-new-ai-biotech-isomorphic-labs/